Landslides are among the most destructive natural hazards, causing significant loss of life and property worldwide, often triggered by intense rainfall. Effective planning and early warning systems (EWS) can dramatically reduce their impact, potentially preventing over 90% of damages.
An EWS integrates susceptibility maps, rainfall thresholds, and real-time meteorological data to predict landslide occurrence. While physically based models suit small-scale applications, empirical approaches are more adaptable to broader regions when historical data is available.
In Vietnam—particularly in mountainous areas like Ha Long City—frequent extreme weather events heighten landslide risk. However, challenges such as sparse landslide data and urbanization complicate the development of reliable systems.
Addressing data limitations is essential to building effective early warning systems for at-risk areas.
Read More: Empirical Landslide Early Warning System: A Case Study from Ha Long City, Vietnam.
Study Area
Quang Ninh, located in the northeast of Vietnam, features diverse topography that includes coastal plains, swamps, midland hills, and mountainous terrain. The region experiences a tropical climate, with hot, humid summers from May to October and cold, dry winters from November to April.
Averaging 1,995 mm of precipitation annually, the majority of rainfall occurs during the summer months (80–85%). Due to a combination of natural, environmental, and societal factors, the mountainous areas of Quang Ninh are particularly prone to natural disasters, especially landslides.
Ha Long, the capital of Quang Ninh, has witnessed rapid growth driven by its coal resources, tourism, and trade. Its diverse topography, including mountains, deltas, coastal areas, and islands, contributes to complex landforms, with the northern and northwest regions covering about 70% of the land area.
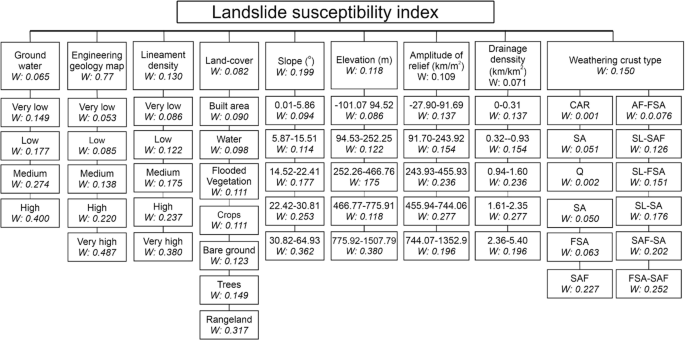
According to the Quang Ninh Department of Construction (DOC 2016), many households in Ha Long City are situated in landslide-prone areas. As such, developing a landslide early warning system for Ha Long City is urgent.
Methodology and Results
Landslide Susceptibility Map Using the Spatial Multi-Criteria Evaluation (SMCE) Method
The Spatial Multi-Criteria Evaluation (SMCE) method is a semi-quantitative technique used to assess landslide susceptibility by integrating various spatial criteria.
Developed from the Analytical Hierarchical Process (AHP), this method facilitates multi-criteria analysis in spatial models and addresses the limitations of purely quantitative approaches, which often require extensive and comprehensive data.
SMCE relies heavily on expert judgment, as each causal factor contributing to landslides is evaluated based on expert experience, particularly when numeric data is limited or unavailable.SMCE’s input maps serve as spatial criteria, which are normalized, weighted, and grouped into a “criteria tree.”
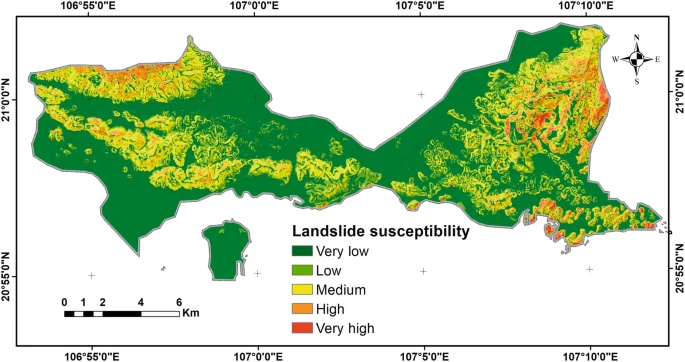
The output is one or more composite index maps that represent simulated results. The process begins by collecting data and spatial maps, followed by normalization, rasterization, and classification of spatial factors.
The criteria are structured into a tree, with weights assigned to each factor based on expert judgment using tools like pairwise comparison and ranking. The final output is the Landslide Susceptibility Index (LSI) map, which is classified into five susceptibility classes: Very low, low, moderate, high, and very high.
For Quang Ninh, nine primary factors, including elevation, slope, land cover, relief amplitude, lineament density, drainage density, groundwater availability, and weathering crust type, were chosen as inputs.
These factors were obtained from the State-Funded Landslide Project (SFLP) at various scales and processed using ArcGIS software.The analysis confirmed the role of these factors in landslide susceptibility, based on expert assessments from field surveyors and research staff.
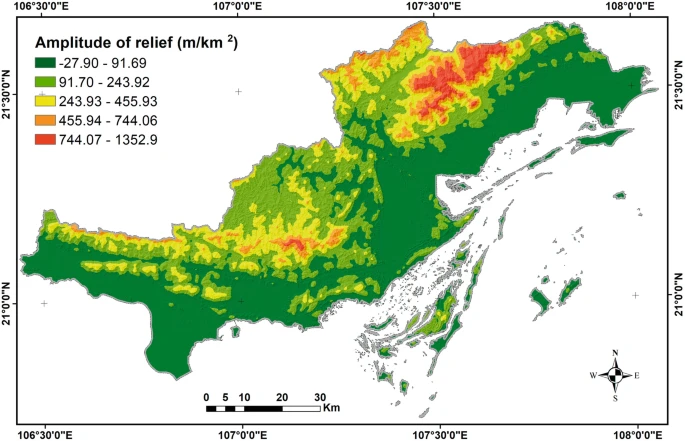
The LSI map generated for Quang Ninh, particularly Ha Long City, was validated by comparing the predicted susceptibility zones with observed landslide locations.
Results showed that 63% of recorded landslides occurred in zones with high or very high susceptibility, despite these zones covering only 29% of the total area. In Ha Long City, 40% of observed landslides occurred in the two most susceptible zones, covering just 8% of the city’s area.
Empirical Rainfall Threshold
The concept of Empirical Rainfall Threshold is a critical method used in hydrology and geotechnical engineering to predict landslide occurrences based on rainfall data.
This approach relies on the premise that specific rainfall conditions, such as the intensity, duration, and accumulation of rainfall, can serve as indicators for the initiation of landslides.
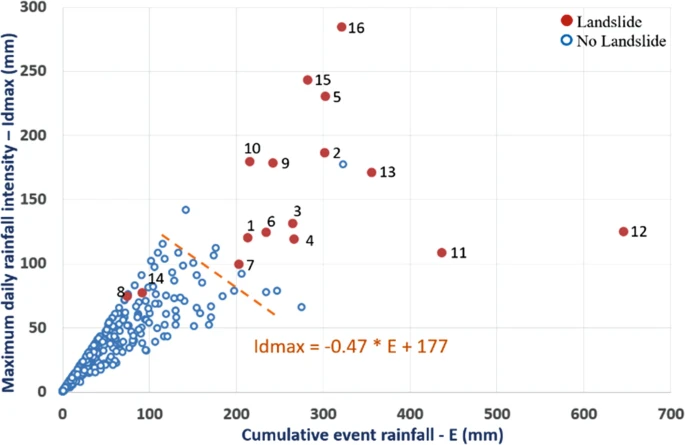
By examining historical rainfall data, researchers can establish a threshold, or a set of values, that once exceeded, significantly increases the likelihood of a landslide event.
The empirical method is grounded in past observations and relationships between rainfall events and landslide occurrences, meaning that it assumes patterns from previous events will repeat under similar conditions.
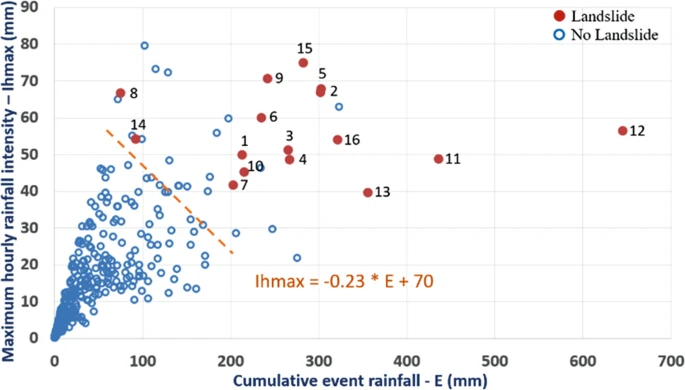
Typically, this method involves analyzing hourly and daily rainfall data, focusing on factors such as the maximum rainfall intensity, cumulative rainfall over several days, and antecedent rainfall, which collectively influence the soil’s ability to withstand the pressure and weight of additional moisture.
This threshold, once established, can be applied to forecast the likelihood of future landslides, aiding in risk management and early warning systems.
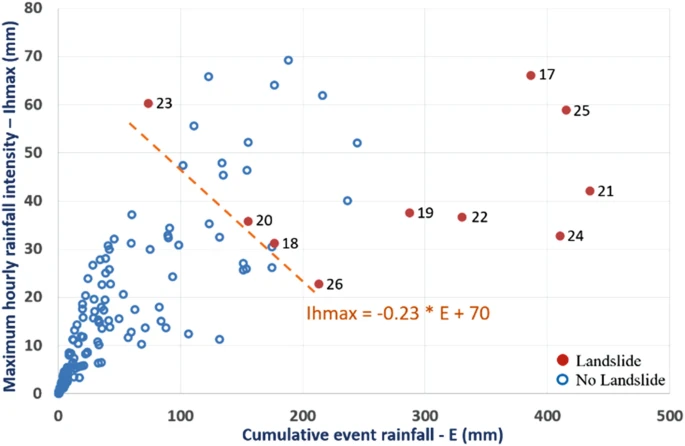
Through careful data collection and statistical analysis, empirical rainfall thresholds help mitigate the devastating impacts of landslides, particularly in areas prone to frequent heavy rainfall.
Evaluation of Rainfall Thresholds for Landslide Prediction
During the test period, out of 190 rainfall events, 17 surpassed the threshold Td, but 9 of these events (52.9%) did not result in landslides. This implies that although these events met the rainfall threshold, they were not linked to landslides.
On the other hand, when the Td threshold was applied, 8 out of 10 rain-induced landslides (80%) were appropriately detected and could be warned in advance.
However, when threshold Th was utilized, the number of rainfall events that surpassed the threshold but were not associated with landslides increased to 56.5% (13 out of 23 events).
Despite this, using threshold Th in the early warning system resulted in a safer outcome, with a 100% success rate in forecasting all rainfall-triggered landslide events.In evaluating the effectiveness of the two thresholds, four potential outcomes (Staley et al., 2012) were considered.
These include: a True Positive (TP), where the data point exceeds the rainfall threshold and a landslide occurs; a True Negative (TN), where the data point is below the threshold and no landslide occurs; a False Positive (FP).
Where the data point exceeds the threshold but no landslide occurs; and a False Negative (FN), where the data point is below the threshold but a landslide is recorded.
To assess the performance of the Td and Th thresholds, several skill scores were calculated based on common accuracy evaluation methods (Segoni et al., 2018; Staley et al., 2012).
These included: the Probability of Detection (POD), which measures the proportion of correct warnings for landslides; the Probability of False Detection (POFD), indicating how often a landslide is incorrectly predicted; the Probability of False Alarm (POFA).
Showing how often a warning is given without a corresponding landslide; and the Hanssen and Kuipers (HK) Skill Score, which combines POD and POFD to determine the overall accuracy of the prediction.
Proposed Landslide Early Warning System
The Proposed Landslide Early Warning System aims to provide timely and accurate alerts for potential landslide occurrences triggered by rainfall events. By utilizing rainfall thresholds, such as the refined threshold Th, the system will analyze real-time weather data to predict the likelihood of landslides.
This system incorporates key variables like maximum hourly and daily rainfall, antecedent rainfall, and cumulative event rainfall to assess the risk of landslides in high-risk areas.
With the threshold Th offering a higher accuracy rate in predicting rainfall-triggered landslides, the early warning system will enable authorities and residents in vulnerable areas to take proactive measures, such as evacuations or road closures, to minimize the impact of landslides.
By integrating data from local hydro-meteorological stations and continuously updating thresholds based on ongoing rainfall patterns, this system promises to enhance public safety and disaster preparedness in landslide-prone regions.
Frequently Asked Questions (FAQs)
What is a Landslide Early Warning System?
A Landslide Early Warning System (LEWS) is a technology-driven system designed to provide timely alerts about the likelihood of landslides triggered by specific environmental conditions, such as heavy rainfall. It uses real-time data, such as rainfall thresholds, to predict and warn about potential landslide events, helping authorities and residents take preventive measures to reduce risks.
How does the Landslide Early Warning System work?
The system monitors rainfall data, specifically hourly and daily rainfall, as well as antecedent rainfall, and compares it with pre-established thresholds that have been linked to past landslide events. When rainfall exceeds certain thresholds, the system generates a warning to notify stakeholders about potential landslides, allowing them to take action before an event occurs.
Why is the threshold Th recommended for the warning system?
The threshold Th has shown higher accuracy in predicting rainfall-induced landslides compared to other thresholds. It produces fewer false alarms while maintaining a high probability of detecting actual landslide events. This makes it a safer and more reliable option for early warning systems, as it ensures that nearly 100% of rainfall-triggered landslides are predicted.
What are the key variables used in the warning system?
Key variables include maximum hourly rainfall, maximum daily rainfall, cumulative event rainfall, and antecedent rainfall over various time durations. These data points are crucial for determining whether conditions are favorable for landslide initiation and trigger the early warning system accordingly.
How can the Landslide Early Warning System help communities?
The system helps protect communities by providing early warnings of impending landslides, allowing local authorities to take preventive actions, such as evacuations, road closures, or temporary shutdowns of critical infrastructure. This minimizes property damage and loss of life during landslide events.
Can the Landslide Early Warning System be used in all regions?
While the system can be implemented in landslide-prone areas with access to rainfall data and other environmental conditions, its effectiveness depends on the accuracy of the threshold data and local conditions. For optimal results, thresholds should be regularly updated and tailored to the specific geography and rainfall patterns of the region.
Conclusion
The proposed Landslide Early Warning System (LEWS) represents a significant advancement in disaster risk management, particularly in landslide-prone areas such as Ha Long City. By utilizing precise rainfall thresholds, the system can accurately predict and warn of potential landslides, enabling timely intervention and reducing the risk of fatalities and property damage.